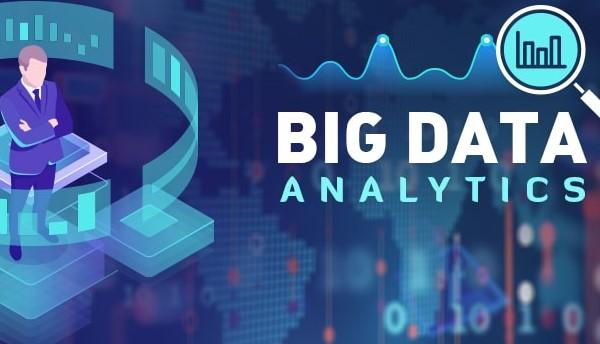
Big data analytics involves the process of analyzing raw data collected from different sources and using it to collect vital insights that are later incorporated into the business operations to give direction on the way forward. The insights aid in identifying the best time possible to roll a new product, and structure an effective campaign that will be used to transform the business from one level to the next.
It’s evident that big data analytics is the primary driver of any business that has excellent success plans in the future. However, the riddle remains on how data professionals use this technology to convert data into meaningful insights that the business stakeholders can utilize in determining a bright future for the respective business. Many people do not know that there are different methods and techniques applied in data processing to generate insights.
Remember that approximately 90% of the data processed during this methodology is mainly unstructured in nature. Big data analytics techniques play an integral role in processing and analyzing crucial data elements and generate valuable data insights that give the business a conducive platform where it can facilitate its daily operations. However, during data processing, you need to understand that two types of data are processed to generate insights. They include:
Quantitative Data
Quantitative data refers to any kind of data that can be measurable (quantifiable) in nature. It mainly consists of specific quantities such as numeric numbers, the number of website visitors, and sales figures, among the rest. This kind of data is easy to process and generates insights given that it can easily be read and understood by machines during processing.
Qualitative Data
Qualitative data is the opposite of quantitative data since it cannot be measured or quantified. Some of these data include responses left by customers after reading a particular survey, the text within product reviews, and what people say during interviews. This kind of information cannot be measured making it hard to process and generate insights. In real-life applications, qualitative data may be audio transcriptions or video clips stored on a commercial surveillance system.
To be able to analyze data, you need to incorporate lots of strategies such as analytics and reports to collect enough information that you can apply in your business operations. This article incorporates a detailed guide on some of the best big data analytics techniques that all data professionals and business stakeholders need to know. Let’s get started!
1. Regression Analysis
This is a technique that is applied to estimate the relationship between different sets of variables. When working on anything to do with regression analysis, you simply focus on identifying any possible relationship existing between various variables within your data sets. At this point, you deal with both the dependent and the independent data variables. The two works together to help you make proper predictions that will contribute to your business’s success in the long run.
For instance, when working within the e-commerce sector, you may want to identify the relationship between the amount of money you spent on social media marketing and the revenue you generate from the marketing efforts. In this case, you need to utilize regression analysis to analyze both the independent and the dependent variables to uncover insights that will help you come to an incredible solution.
2. Factor Analysis
Factor analysis is applied when you want to reduce a considerable number of variables into smaller bits for ease of processing. This technique mainly operates by considering that multiple separate, observable variables correlate because they operate in the same underlying construct. Note that factor analysis is not only necessary because it reduces massive numbers to smaller variables but also aids in discovering data patterns and trends.
Once this is done, data professionals can easily identify hidden data insights that cannot be easily measured or even observed when a manual processing methodology is applied. Using this technique allows you to analyze and measure data concepts that cannot be observed and even measured. This includes happiness, emotions, and fitness, among others.
3. Cluster Analysis
Cluster analysis is also considered an experimental technique mainly focused on uncovering structures within a given data set. The ultimate goal of this strategy is to sort different data elements into different groups depending on the nature of the data. The data with similar characteristics are sorted into similar clusters. However, every cluster is different from the others based on the data that has been classified. In addition, clustering gives you a clear picture of how data is organized into various groups during the analytics process.
This data analytics technique is commonly used within business settings to sort between different customers to help the business understand what a specific group wants. Also, the strategy can be applied to evaluate business performance, focusing on demographic factors since it’s pretty easy to classify various demographics into different categories and analyze their needs at a personal level. However, even though this strategy may uncover structures in your data, it does not give more explanation and reasons why they exist.
4. Sentiment Analysis
Once data is mentioned, most people will automatically think of numbers and spreadsheets. Most company stakeholders forget to evaluate qualitative data since they do not know its value in data analytics. The truth is that qualitative data comes with a lot of insights that can help you understand the nature of your customers. Sentiment analysis aids in analyzing data in a much broader view. This technique can help uncover insights based on emotions outlined in a text format.
If you utilize this technique in analyzing your qualitative data, you will learn how customers perceive your brand, especially the products and services you offer. This will give you an added advantage since you can now tailor your products and services in a manner that intrigues your customers, increasing your chances of making more sales and generating more revenue.
5. Time Series Analysis
This data analytics technique is tailored toward identifying trends and patterns over a particular period. The time-series analytics is crafted to measure a sequence of data points at different times, depending on your preference. You can decide to measure daily, weekly, monthly, or even year-over-year growth to evaluate the business performance. Considering trends and patterns based on time allows the business stakeholders to monitor the milestones attained within a particular period.
Once you have decided to conduct a time series analysis, you need to evaluate things such as seasonality, cyclic patterns, and market trends. These features are mainly applied to give you a clear viewing point that will likely impact your business performance.
Conclusion
Big data analytics significantly impacts the well-being of companies across industries. Even though 67% of companies have their infrastructure housed in the cloud, only a few companies utilize the provided tools and techniques in their data operations. There is a need for businesses to capitalize on the big data analytics opportunities they have to propel their performance to the next level.